What is Research Bias - Types & Examples
Research is crucial in generating knowledge and understanding the world around us. However, the validity and reliability of research findings can be compromised by various factors, including bias in research. This comprehensive guide will explain the different examples and types of research bias. But before that, let’s look into the research bias definition.
What is Research Bias?
Research bias refers to the systematic errors or deviations from the truth that can occur during the research process, leading to inaccurate or misleading results. It arises from flaws in the research design, data collection, analysis, and interpretation, which can distort the findings and conclusions. Bias in research can occur at any stage of the research process and may be unintentional or deliberate. Recognising and addressing research bias is crucial for maintaining the integrity and credibility of scientific research.
Example of Bias in Research
Suppose a researcher wants to investigate the relationship between coffee consumption and heart disease risk. They recruit participants for their study and ask them to self-report their coffee intake through a questionnaire. Bias can occur in this scenario due to self-reporting bias, where participants may provide inaccurate or biased information about their coffee consumption.
For example, health-conscious individuals might underreport their coffee intake because they perceive it as unhealthy, while coffee enthusiasts might overreport their consumption due to their positive attitude towards coffee.
Types of Research Bias
There are many different types of research bias. Some of them are discussed below.
- Information Bias
- Publication Bias
- Interviewer Bias
- Response Bias
- Researcher Bias
- Selection Bias
- Cognitive Bias
Information Bias
Information bias is also known as measurement bias. It refers to a type of research bias that occurs when there are errors or distortions in gathering, interpreting, or reporting information in a research study or any other form of data collection.
Example of Information Bias In Research
Let's say you are studying the effectiveness of a new weight loss program. You recruit participants and ask them to keep a daily food diary to track their caloric intake. However, the participants know that they are being monitored and may alter their eating habits, consciously or unconsciously, to present a more favourable image of themselves.
In this case, the participants' awareness of being observed can lead to information bias in research. They might underreport their consumption of high-calorie foods or overreport their consumption of healthy foods, skewing the data collected. This research bias could make the weight loss program appear more effective than it actually is because the reported dietary intake doesn't accurately reflect the participants' true behaviour.
Types of Information Bias In Research
Information bias can manifest in different ways, such as:
1. Measurement Bias
Measurement Bias occurs when the measurement instruments or techniques used to collect data are flawed or inaccurate. For example, if a survey question is poorly worded or ambiguous, it may generate biased responses or misinterpretations of the respondents' answers.
2. Recall Bias
Recall bias arises when participants in a study inaccurately remember or recall past events, experiences, or behaviours. It can happen due to various factors, such as selective memory, social desirability bias, or the passage of time. Recall bias causes distorted or unreliable data.
3. Reporting Bias
Reporting bias occurs when there is selective or incomplete reporting of study findings. It can happen if researchers or organisations only publish or publicise results that support their preconceived notions or desired outcomes while omitting or downplaying contradictory or unfavourable findings. Reporting bias can lead to a skewed perception of the true state of knowledge in a particular field.
4. Publication Bias
Publication bias refers to the tendency of researchers, journals, or other publishing entities to publish studies with statistically significant or positive results preferentially. Studies with null or negative findings are often less likely to be published, leading to an overrepresentation of positive results in the literature and potentially distorting the overall understanding of a research topic.
5. Language Bias
This bias can transpire if research is conducted and reported in a specific language, leading to limited accessibility and potential exclusion of relevant studies or data published in other languages. Language bias can introduce distortions in systematic reviews, meta-analyses, or other forms of evidence synthesis.
Publication Bias
Publication bias occurs due to the systematic tendency of scientific journals and researchers to preferentially publish studies with positive or significant results while overlooking or rejecting studies with negative or non-significant findings. It transpires when the decision to publish a study is influenced by the nature or direction of its results rather than its methodological rigour or scientific merit.
Publication bias in research can arise due to various factors, such as researchers' and journals' preferences for novel or groundbreaking findings, the pressure to present positive results to secure funding or advance academic careers, and the tendency of studies with positive results to generate more attention and citations. This research bias can distort the overall body of scientific literature, leading to an overrepresentation of studies with positive outcomes and an underrepresentation of studies with negative or inconclusive findings.
Example of Publication Bias In Research
Let's say a pharmaceutical company conducts a clinical trial to test the effectiveness of a new drug for treating a certain medical condition. The company conducts several trials but only submits the results of the trials that show positive outcomes that states that the drug is effective to scientific journals for publication, as the negative results may lead to rejection in funding.
Interviewer Bias
Interviewer bias means the potential for bias or prejudice to influence the outcome of an interview. It happens when the interviewer's personal beliefs, preferences, stereotypes, or prejudices affect their evaluation of the interviewee's qualifications, skills, or suitability for a position.
Example of Interviewer Bias In Research
Imagine there is an interviewer named James conducting interviews for a sales position in a company. During one interview, a candidate named Aisha, who is a woman, showcases exceptional knowledge about the products, demonstrates excellent communication skills, and presents a strong sales track record.
However, James thinks women are generally less assertive or aggressive in sales roles than men. Due to this stereotype bias in research, James may subconsciously underestimate Aisha's abilities or question her suitability for the position, despite her impressive qualifications.
Types of Interviewer Bias In Research
The main types of interviewer bias are:
1. Stereotyping
Stereotyping refers to holding preconceived notions or stereotypes about certain groups of people based on their race, gender, age, religion, or other characteristics. These biases can lead to unfair judgments or assumptions about the interviewee's abilities.
2. Confirmation Bias
In confirmation bias, Interviewers may subconsciously seek information that confirms their pre-existing beliefs or initial impressions about the interviewee. This results in selectively noticing and emphasising certain responses or behaviours that align with their biases while disregarding contradictory evidence.
3. Similarity Bias
Similarity bias means unconsciously favouring candidates with similar backgrounds, experiences, or characteristics, resulting in a preference for more relatable or familiar candidates. This leads to overlooking qualified candidates from diverse backgrounds.
4. Halo and Horns Effect
The halo effect occurs when an interviewer forms an overall positive impression of a candidate based on one favourable characteristic, leading to a bias in favour of that candidate. Conversely, the horns effect occurs when a negative impression of a candidate's single attribute influences the overall evaluation, resulting in a bias against the candidate.
5. Contrast Effect
The contrast effect leads to evaluating candidates relative to each other rather than based on objective criteria, leading to biased judgments. If the previous candidate was exceptionally strong or weak, the current candidate might be evaluated more harshly or leniently.
6. Implicit Bias
Interviewers may have unconscious biases influencing their perceptions and decision-making. Societal stereotypes often form these biases and can affect evaluations and decisions without the interviewer's conscious awareness.
Response Bias
Response bias arises from a systematic error or distortion in how individuals respond to survey questions or provide information in research studies. It occurs when respondents consistently tend to answer questions inaccurately or in a particular direction, leading to a skewed or biased dataset.
Example of Response Bias In Research
You conduct a survey asking people about their exercise habits and distribute the survey to a group of individuals. You ask them to report the number of times they exercise per week. However, some respondents may feel pressured to provide answers they believe are more socially acceptable. They might overstate their exercise frequency to present themselves as more active and health-conscious. This would result in an overestimation of exercise habits in the data.
Types of Response Bias In Research
We have discussed a few common types of response bias below. Other major types include courtesy bias and extreme responding.
1. Social Desirability Bias
This occurs when respondents provide answers that they perceive to be more socially acceptable or desirable than their true beliefs or behaviours. They may modify their responses to conform to societal norms or present themselves favourably.
2. Acquiescence Bias
Also known as "yea-saying" or "nay-saying," Acquiescence bias in research is the tendency of respondents to agree or disagree with statements or questions without carefully considering their content. Some individuals are predisposed to consistently agree (acquiesce) or disagree with items, leading to skewed responses.
3. Non-Response Bias
This bias emerges when individuals who choose not to participate in a study or survey have different characteristics or opinions compared to those who do participate.
Researcher Bias
Researcher bias, also known as experimenter bias or investigator bias, refers to the influence or distortion of research findings or interpretations due to the personal beliefs, preferences, or expectations of the researcher conducting the study. It occurs when the researcher's subjective biases or preconceived notions unconsciously affect the research process, leading to flawed or biased results.
Example of Researcher Bias In Research
Assume that a researcher is conducting a study on the effectiveness of a new teaching method for improving student performance in mathematics. The researcher strongly believes the new teaching method will significantly enhance students' mathematical abilities.
To test the method, the researcher divides students into two groups: the control group, which receives traditional teaching methods, and the experimental group, which receives the new teaching method.
During the study, the researcher spends more time interacting with the experimental group, providing additional support and encouragement. They unintentionally convey their enthusiasm for the new teaching method to the students in the experimental group while giving a different level of attention or encouragement to the control group.
When the post-test results come in, the experimental group shows a statistically significant improvement in mathematical performance compared to the control group. Influenced by their initial beliefs and unintentional differential treatment, the researcher concludes that the new teaching method is highly effective in enhancing students' mathematical abilities.
Hire an Expert Writer
Proposal and research paper orders completed by our expert writers are
- Formally drafted in academic style
- Plagiarism free
- 100% Confidential
- Never Resold
- Include unlimited free revisions
- Completed to match exact client requirements
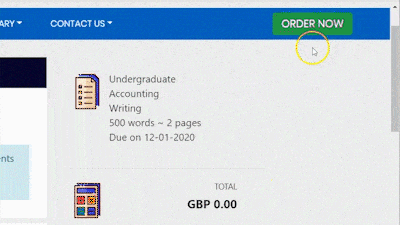
Selection Bias
Selection bias refers to a systematic error or distortion that occurs in a research study when the participants or subjects included in the study are not representative of the target population. This research bias arises when the process of selecting participants for the study is flawed or biased in some way, leading to a sample that does not accurately reflect the characteristics of the broader population.
Example of Selection Bias In Research
Suppose a research team wants to evaluate the weight loss program's effectiveness and recruits participants by placing an advertisement in a fitness magazine. The advertisement attracts health-conscious individuals who are actively seeking ways to lose weight. As a result, the study sample primarily consists of highly motivated individuals to lose weight and may have already tried other weight loss methods.
The sample is biased towards individuals more likely to succeed in weight loss due to their pre-existing motivation and experience.
Types of Selection Bias In Research
Selection bias can occur in various forms and impact both observational and experimental studies. Some common types of selection bias include:
1. Non-Response Bias
This occurs when individuals chosen for the study do not participate or respond, leading to a sample that differs from the target population. Non-response bias can introduce bias in research if those who choose not to participate have different characteristics from those who do participate.
2. Volunteer Bias
Volunteer bias happens when participants self-select or volunteer to participate in a study. This can lead to a sample not representative of the broader population because volunteers may have different characteristics, motivations, or experiences compared to those who do not volunteer.
3. Healthy User Bias
This research bias can occur in studies that examine the effects of a particular intervention or treatment. It arises when participants who follow a certain lifestyle or treatment regimen are healthier or have better health outcomes than the general population, leading to overestimating the treatment's effectiveness.
4. Berkson's Bias
This research bias occurs in hospital-based studies where patients are selected based on hospital admission. Since hospital-based studies typically exclude healthy individuals, the sample may consist of patients with multiple conditions or diseases, leading to an artificial association between certain variables.
5. Survivorship Bias
Survivorship bias happens when the sample includes only individuals or entities that have survived a particular process or undergone a specific experience. This bias can lead to an inaccurate understanding of the entire population since it neglects those who did not survive or dropped out.
Cognitive Bias
A cognitive bias refers to systematic patterns of deviation from rational judgment or decision-making processes, often influenced by subjective factors and unconscious mental processes. These research biases can affect how we interpret information, judge, and form beliefs. Cognitive biases can be thought of as shortcuts or mental filters that our brains use to simplify complex information processing.
Example of Cognitive Bias In Research
Assume that you are investigating the effects of a new drug on a particular medical condition. Due to prior experiences or personal beliefs, the researcher has a positive view of the drug's effectiveness. During the research process, the researcher may unconsciously focus on collecting and analysing data that supports their preconceived notion of the drug's efficacy. They may pay less attention to data that suggests the drug has limited or no impact.
Types of Cognitive Bias In Research
Some of the most common types of cognitive bias are discussed below.
1. Confirmation Bias
The tendency to seek, interpret, or remember information in a way that confirms one's existing beliefs or hypotheses while disregarding or downplaying contradictory evidence.
2. Availability Heuristic
This research bias occurs when you overestimate the importance or likelihood of events based on how easily they come to mind or how vividly they are remembered.
3. Anchoring Bias
Relying too heavily on the first piece of information encountered (the "anchor) when making decisions or estimations, even if it is irrelevant or misleading.
4. Halo Effect
The halo effect happens when you generalise positive or negative impressions of a person, company, or brand based on a single characteristic or initial experience.
5. Overconfidence Effect
The tendency to overestimate one's abilities, knowledge, or the accuracy of one's beliefs and predictions.
6. Bandwagon Effect
Preferencing to adopt certain beliefs or behaviours because others are doing so, often without critical evaluation or independent thinking.
7. Framing Effect
The framing effect refers to how the information presented or "framed" can influence decision-making, emphasising the potential gains or losses, leading to different choices even when the options are objectively the same.
How to Avoid Research Bias?
Avoiding research bias is crucial for maintaining the integrity and validity of your research findings. Here are some strategies on how to minimise research bias:
- Formulate a clear and specific research question that outlines the objective of your study. This will help you stay focused and reduce the chances of introducing research bias.
- Perform a thorough literature review on your topic before starting your research. This will help you understand the current state of knowledge and identify potential biases or gaps in the existing research.
- Use randomisation and blinding techniques to ensure that participants or samples are assigned to groups unbiasedly. Blinding techniques, such as single-blind or double-blind procedures, can be used to prevent bias in data collection and analysis.
- Ensure that your sample is representative of the target population by using random or stratified sampling methods. Avoid selecting participants based on convenience, as it can introduce selection bias.
- Consider using random invitations or incentives to encourage a diverse range of participants.
- Clearly define and document the methods and procedures used for data collection to ensure consistency. This includes using standardised measurement tools, following specific protocols, and training research assistants to minimise variability and observer bias.
- Researchers can unintentionally introduce bias through preconceived notions, beliefs, or expectations. Be conscious of your biases and regularly reflect on how they influence your research process and interpretation of results.
- Relying on a single source can introduce bias. Triangulate your findings by using multiple methods (quantitative and qualitative) and collecting data from diverse sources to ensure a more comprehensive and balanced perspective.
- Use appropriate statistical techniques and avoid cherry-picking results that support your hypothesis. Be transparent about the limitations and uncertainties in your findings.
Frequently Asked Questions
Bias in research refers to systematic errors or preferences that can distort the results or conclusions of a study, leading to inaccuracies or unfairness due to factors such as sampling, measurement, or interpretation.
Bias in research can be caused by various factors, such as the selection of participants, flawed study design, inadequate sampling methods, researcher's own beliefs or preferences, funding sources, publication bias, or the omission or manipulation of data.
To avoid research bias, use random and representative sampling, blinding techniques, pre-registering hypotheses, conducting rigorous peer review, disclosing conflicts of interest, and promoting transparency in data collection and analysis.
You can critically examine your biases, use diverse and inclusive samples, employ appropriate statistical methods, conduct robust sensitivity analyses, encourage replication studies, and engage in open dialogue about potential biases in your findings.